Damien LaRocque
Proprioception Is All You Need: Terrain Classification for Boreal Forests
My paper, Proprioception Is All You Need: Terrain Classification for Boreal Forests, will be presented in the 2024 IEEE/RSJ International Conference on Intelligent Robots and Systems (IROS 2024), in Abu Dhabi, UAE. The paper presents BorealTC : a publicly available dataset containing annotated data from a wheeled UGV for various mobility-impeding terrain types typical of the boreal forest. The data was acquired in winter and spring on deep snow and silty loam, two uncommon terrains in an urban setting.
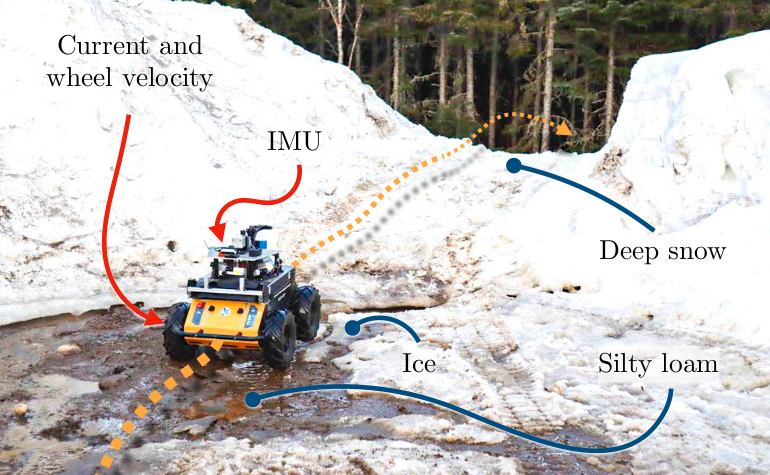
My paper, Proprioception Is All You Need: Terrain Classification for Boreal Forests, will be presented in the 2024 IEEE/RSJ International Conference on Intelligent Robots and Systems (IROS 2024), in Abu Dhabi, UAE. The paper presents BorealTC : a publicly available dataset containing annotated data from a wheeled UGV for various mobility-impeding terrain types typical of the boreal forest. The data was acquired in winter and spring on deep snow and silty loam, two uncommon terrains in an urban setting.
Here is the abstract:
Recent works in field robotics highlighted the importance of resiliency against different types of terrains. Boreal forests, in particular, are home to many mobility-impeding terrains that should be considered for off-road autonomous navigation. Also, being one of the largest land biomes on Earth, boreal forests are an area where autonomous vehicles are expected to become increasingly common. In this paper, we address the issue of classifying boreal terrains by introducing BorealTC, a publicly available dataset for proprioceptive-based terrain classification (TC). Recorded with a Husky A200, our dataset contains 116 min of Inertial Measurement Unit (IMU), motor current, and wheel odometry data, focusing on typical boreal forest terrains, notably snow, ice, and silty loam. Combining our dataset with another dataset from the literature, we evaluate both a Convolutional Neural Network (CNN) and the novel state space model (SSM)-based Mamba architecture on a TC task. We show that while CNN outperforms Mamba on each separate dataset, Mamba achieves greater accuracy when trained on a combination of both. In addition, we demonstrate that Mamba’s learning capacity is greater than a CNN for increasing amounts of data. We show that the combination of two TC datasets yields a latent space that can be interpreted with the properties of the terrains. We also discuss the implications of merging datasets on classification. Our source code and dataset are publicly available online: https://github.com/norlab-ulaval/BorealTC.
Terrains
For this paper, a ClearPath Husky A200 was driven on 5 different terrains:
Links
For more info,
Squirrels
Eichhörnchen
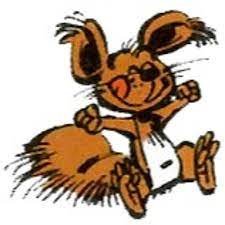
When I talked about my new website to my friend William Guimont-Martin, he immediately had one thing in mind:
So, here are my best squirrel photos. They were taken on May 20th, 2024, in Marais du Nord (46°57'35"N 71°23'35"W).
Hello, World!
Unofficially starting my own website
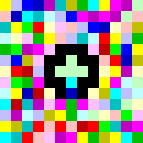
Welcome on my site ! Here, I plan to regularly publish posts about programming, robotics, and electronics. I also plan to publish non-technical content.
In the meantime, you can subscribe to my RSS feed, or you can follow me on these platforms:
- GitHub : IamPhytan
- GitLab : damienlarocque
- LinkedIn : Damien LaRocque
- Twitter : @HistoriCode97
- Mastodon : @historicode97@fosstodon.org
- Send me an email : phicoltan@gmail.com